Next: Conclusions
Up: Poisson background on the
Previous: Inferring
The histograms of
Fig. 11 show examples of the probability
distributions of
for
and three different
hypotheses for
.
Figure:
Inference about
(histograms)
and
(continuous lines)
for
and
,
assuming
and three values of
: 0.75, 0.25 and 0.95 (top down).
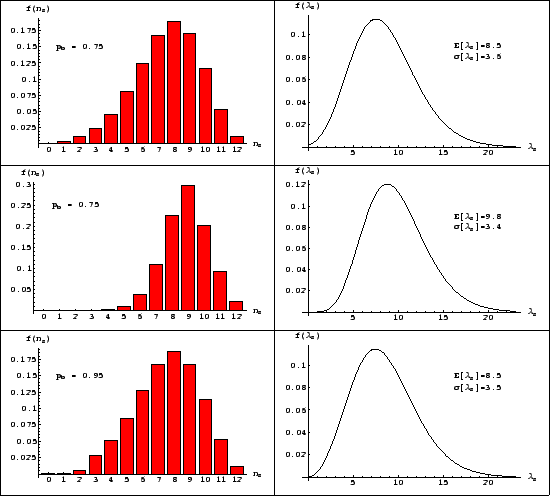 |
These distributions quantify how much we believe that
out of the observed
belong to the signal.
[By the way, the number
of background objects
present in the data can be inferred as complement
to
, since the two numbers are linearly dependent. It follows
that
.]
A different question is to infer the the Poisson
of the signal. Using once more Bayes theorem we get,
under the hypothesis of
signal objects:
Assuming a uniform prior for
we get
(see e.g. Ref. [2]):
with expected value and variance both equal to
and mode equal to
(the expected value is shifted
on the right side of the mode because the distribution is skewed
to the right).
Figure 12
shows these pdf's, for
ranging from 0 to 12 and
assuming a uniform prior for
.
Figure:
Inference of
depending on the
,
ranging from 0 to 12 (left to right curves).
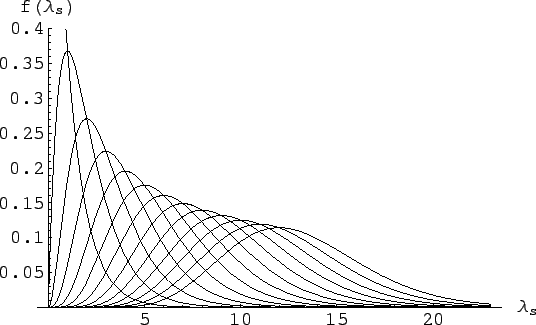 |
As far the pdf of
that depends on all possible
values of
, each with is probability, is concerned,
we get from probability theory
[and remembering that, indeed,
is equal to
, because
depends only on
, and then the other way around]:
i.e. the pdf of
is the weighted
average2
of the several
depending pdf's.
The results for the example we are considering in this
section are given in the plots of Fig. 11.
Next: Conclusions
Up: Poisson background on the
Previous: Inferring
Giulio D'Agostini
2004-12-13