Next: Conclusions
Up: Asymmetric Uncertainties: Sources, Treatment
Previous: Uncertainty due to systematics
Some rules of thumb to unfold probabilistic sensible information
from results published with asymmetric uncertainties
Having understood what one should have done
to obtain expected value and standard deviation
in the situations in which people are used to report
asymmetric uncertainties, we might attempt to recover
those quantities from the published result.
It is possible to do it exactly only if we
know the detailed contributions to the uncertainty,
namely the
or log-likelihood functions of the
so called `statistical terms' and the pairs
, together
to the probabilistic model,
for each `systematic term'.
However, these pieces of information are usually
unavailable. But we can still make some guesses,
based on some rough assumptions, lacking other information:
- asymmetric uncertainties in the `statistical part'
are due to asymmetric
or log-likelihood:
apply corrections given by
Eqs. (15)-(16);
- asymmetric uncertainties in the `systematic part'
comes from nonlinear propagation:
apply corrections given by
Eqs. (28)-(29).
As a numerical example, imagine
we read the
following result (in arbitrary units):
(that somebody would summary as
!).
The only certainty we have, seeing
two asymmetric uncertainties with the same
sign of skewness, is that the result is definitively biased.
Let us try to make our estimate of the bias and
calculate the corrected result (that, not withstanding all uncertainties
about uncertainties, will be closer to the
`truth' than the published one):
- the first contribution gives roughly
[see. Eqs. (15)-(16)]:
- for the second contribution we have
[see. Eqs. (24)-(24),
(28)-(29)]:
Our guessed best result would then
become14
(The exceeding number of digits in the intermediate steps are just
to make numerical comparison with the correct result that
will be given in a while.)
If we had the chance to learn that
the result of Eq. (30) was due
to the asymmetric
fit of
Fig. 2 plus two systematic
corrections, each described by the triangular distribution
of Fig. 1, then we could
calculate expectation and variance exactly:
i.e.
,
quite different from Eq. (30)
and close to the result corrected by
rule of thumb formulae. Indeed, knowing exactly the
ingredients,
we can evaluate
from
Eq.(1) as
although by Monte Carlo.
Figure:
Monte Carlo estimate of the shape of the
p.d.f. of the sum of three independent variables,
one described by the p.d.f. of Fig. 2
and the other two by the
triangular distribution of Fig. 1.
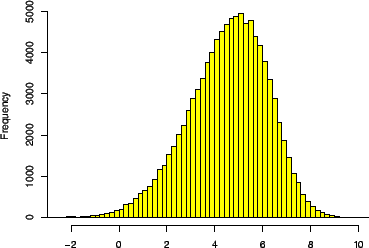 |
The result is given in Fig. 5,
from which we can evaluate a mean value of 4.54 and
a standard deviation of 1.65 in perfect agreement with
the figures given in
Eqs. (37)-(38).15
As we can see from the figure,
also those who like to think at 'best value'
in term of most probable value have to realize once more
that the most probable value of a sum is not
necessarily equal to the sum of most probable values of the
addends
(and analogous statements for all
combinations of uncertainties16).
In the distribution of Fig. 5, the mode of the distribution
is around 5. [Note that expected value and variance are
equal to those given
by Eqs. (37)-(38,
since in the case of a linear combination they can be obtained exactly.]
Other statistical quantities that can
be extracted by the distribution are the median, equal to 4.67,
and some 'quantiles' (values at which the cumulative distribution
reaches a given percent of the maximum -
the median being the 50% quantile). Interesting quantiles
are the 15.85%, 25%, 75% and 84.15%, for which the
Monte Carlo gives the following values of
:
2.88, 3.49, 5.72 and 6.18. From these values we can calculate
the central 50% and 68.3% intervals,17which are
and
, respectively.
Again, the information provided by Eq. (30)
is far from any reasonable way to provide
the uncertainty about
, given the information on each
component.
Besides the lucky case18of this numerical example (which was not constructed
on purpose, but just recycling some material from Ref. [3]),
it seems reasonable that even results roughly corrected
by rule of thumb formulae are already
better than those published directly with asymmetric
result.19
But the accurate analysis can only
be done by the authors
who know the details of the individual contribution to the uncertainty.
Next: Conclusions
Up: Asymmetric Uncertainties: Sources, Treatment
Previous: Uncertainty due to systematics
Giulio D'Agostini
2004-04-27