Effect of the
uncertainties on
and
on the probabilities
of interest
The immediate question that follows is how the uncertainties
concerning these two parameters change the probabilities
of interest. We start reporting in Tab.
Table:
Probability of Infected and Not Infected, given the
test result, as a function of the model parameters.
The third and fourth rows, in boldface,
are for our reference values of
and
. The last
two rows are the results `integrating over' all the possibilities
of
and
, according to
Eq. (
) and
(
),
with the integrals done in practice by Monte Carlo sampling.
|
the dependence of
Inf
Pos
and
NoInf
Neg
, on which we particularly
focused in the previous sections, on the three parameters.
The dependence on
is shown in the different columns, while
the sets of
and
are written explicitly in the
conditionands of the different probabilities.
We start from the nominal values of 0.98 and 0.12 taken from
Ref. [16] (first two rows of the table).
Then we use the expected values calculated
in the previous section (third and fourth rows, in boldface), followed
by variations of
and
based on
one standard deviation
from their expected values.
We see that the probabilities
of interest do not change significantly, the main effect
being due to the assumed proportion of infectees in the
population. One could argue that the dependence on
and
could be larger, if larger deviations
of the parameters were considered.
Obviously this is true, but one has to
take also into account the (small)
probabilities of large deviations
from the mean values, especially if we allow
simultaneous deviations of both parameters.
A more relevant question is, instead, how do
Inf
Pos
and
NoInf
Neg) change, if we take into
account all possible variations of the two parameters
(weighed by their probabilities!).
This is easily done, applying the result of
probability theory that we have already used above.
We get, for the probabilities we are mostly interested in,
Inf Pos |
 |
Inf Pos d d |
(39) |
|
|
|
|
NoInf Neg |
 |
NoInf Neg d d |
(40) |
where
can be factorized into
.22The integral can be easily done by Monte Carlo,23whose implementation in the R language [25], both for
Inf
Pos
and
NoInf
Neg
, is
given in Appendix B.1.
We get, for our arbitrary reference value of
,
Inf
Pos
and
NoInf
Neg
, to be compared
to 0.4858 and 0.9973, respectively, if the expected values were used.
The results, shown with an exaggerated number of digits
just to appreciate tiny differences, are practically the same.
This result could sound counter-intuitive, especially if
one thinks that
has an almost 20% intrinsic
standard uncertainty.
The reason is due to the fact that the dependence of the
probabilities of interest on
and
is rather linear in the region where their probability
mass is concentrated, as shown in Fig.
.
Figure:
Dependence of
Inf
Pos
(upper plots) and
NoInf
Neg
(lower plots)
on
(left hand plots, for
and
) and on
(right hand plots, for
and
). The parameters
and
are
allowed to change withing a range of
's around
their expected values.
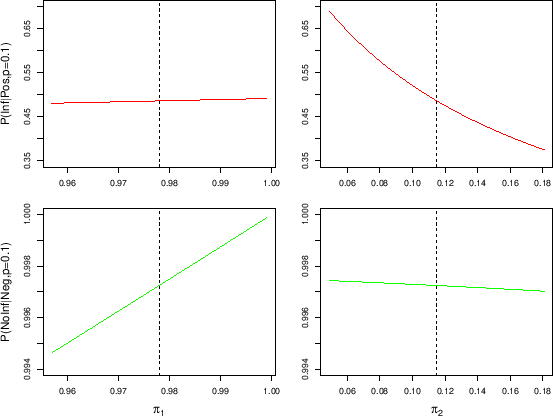 |
This rather good linearity causes a high degree of cancellations
in the integral.24This explains why the only perceptible effect appears
in
Inf
Pos
, slightly
larger than the number calculated at the expected values
(49.04% vs 48.58%), caused by the small non-linearity
of that probability as a function of
,
as shown in the upper, right hand plot of
Fig.
: symmetric
variations of
cause slightly
asymmetric variations of
Inf
Pos
,
thus slightly favoring higher
values of that probability.