Inferred distribution of
Let us start with pdf of
. Our starting point is
from which it follows
in which we have explicitly factorized
.
Again, besides
, we recognize in the integrand
something proportional to a Gamma pdf. If we then
model also
by a Gamma of parameters
and
,
and again neglect
irrelevant factors, we get
Indicating, in analogy to what done to obtain
Eq. (
),
the power of
as
, and the factor multiplying
at the exponent
as
, we get
What is interesting with this result is that we can
consider the term inside the square brackets as an effective likelihood
(remember that multiplicative factors are irrelevant),
and therefore we can rewrite Eq. (
) as
For this reason we can serenely proceed assuming
a flat prior about
, because we can reshape
in a second step the result (see Ref. [1] for details).
So, assuming
and comparing the expression inside the square bracket
of Eq. (
)
with
Eq. (
) we get
the normalization just by analogy, thus getting
or
that, for a flat prior about
, i.e.
and
, becomes
The comparison of this result with
Eq. (
),
obtained using flat priors for
and
,
is at least surprising: the structures of the pdf's are the same,
but
in Eq. (
)
is replaced by
in Eq. (
).
Obviously, the two results will coincide for large
,
and also for small
there is not a dramatic
difference, as we can see from Fig.
.
Figure:
Dependence of the inference of
from the
priors. Solid lines: flat priors on
and
, as in
Figs.
and
,
following from the causal model depicted in Fig.
.
Dashed lines: flat priors on
and
(causal model of Fig.
.)
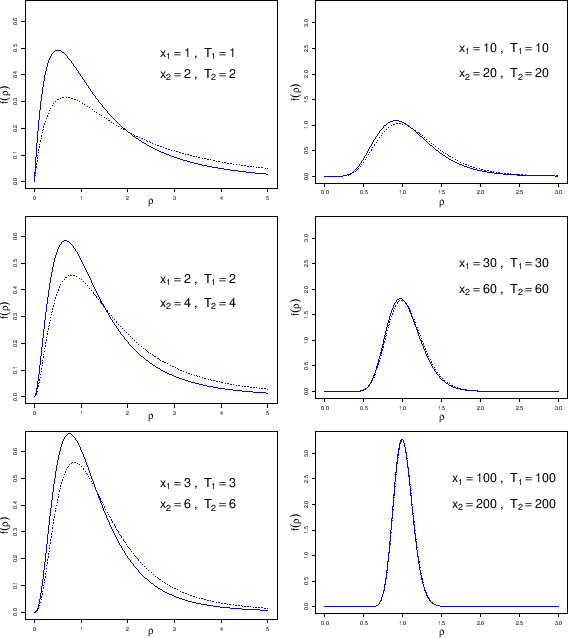 |
As far as the summaries of the distribution are concerned, we get
At this point, instead of taking comfort for the fact that
the differences are irrelevant in practical cases,
or tout court `rejecting Bayesian methods because
of their dependence of priors', it is interesting to try
to understand the origin of this effect,
certainly related to the priors.
But, before proceeding, let
us not forget that Eq. (
)
was obtained
assuming a flat prior about
and that in that model
this prior can be factorized.
Therefore the more general pdf of the rate ratio for the model of
Fig.
is
having only the limitation (but in reality almost irrelevant, given
the flexibility of the Gamma distribution) of depending
on the chosen parametrization for
.