It is rather obvious to think that, repeating the same test with
samples of exactly the same size, but involving
different individuals, no one would be surprised
to count different numbers of positives and negatives in the two samples.
In fact, sticking for a while only to infectees and
assuming an exact value of
, the number
of positives
is given by the binomial distribution,
that is, in short (with `
' to be read as `follows...'),
The probability distribution (
) describes
how much we have to rationally believe to observe
the possible values of
(integers between 0 and
),
given
and
.
An inverse problem is to infer
, given
and the observed number
(indeed, there is also a second inverse problem, that is inferring
from
and
- the three problems
are represented graphically by the networks
of Fig.
).
Figure:
Graphical models of the binomial
distribution (left) and its `inverse problems'. The symbol
`
' indicates the `observed' nodes
of the network, that is the value of the quantity
associated to it
is (assumed to be) certain. The other node
(only one in this simple case)
is `unobserved' and it is associated to
a quantity whose value is uncertain.
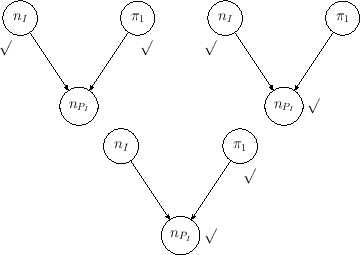 |
This is the kind of
Problem in the Doctrine of Chances first solved by
Bayes [35],
and, independently and in a more refined way, by
Laplace [27] about 250 years ago.
Applying the result of probability theory that
nowadays goes under the name of
Bayes' theorem (or Bayes' rule) that we
have introduced in the previous section, we get, apart from the
normalization factor
hereafter the same generic
symbol is
used for both probability functions and
probability density functions (pdf), being the meaning clear from the
context
:15
where
is the prior pdf, that describes how we believe
in the possible values of
`before' (see
footnote
and Sec.
)
we get the knowledge
of the experiment
resulting into
successes in
trials.
Naively one could say that all possible values of
are equally possible, thus resulting in
.
But this is absolutely unreasonable,16in the case of instrumentation
and procedures devised by experts in order
to hopefully tag infected people as positive.
Therefore the value of
should be most likely
in the region above
, though without sharp cut below it.
Similarly, reasonable values of
are expected
to be in the region below
.