More remarks on the role of priors
Having checked the agreement between the two methods,
let us now focus the attention on the
results themselves.
Looking at the results from the smaller sample we note:
- The width of the distribution using a flat prior
is wider for the small sample than that obtained with larger `statistics',
as expected, with a tiny variation
in the mean value:
.
- The prior
Beta
causes a larger shift of the
distribution towards higher values of
, thus
yielding
.
It is interesting to compare these results with what
we have seen in Sec.
(see Fig.
).
In that case the non-flat, `informative' prior
had the role of `reshaping'
the posterior derived by a flat prior, making
thus the result acceptable by the `expert',
because the outcome was not in contrast with her prior belief.
Here, instead, the result provided
by a flat prior is so far from the rational belief
(most likely shared by the relevant scientific community)
of the expert, that the result would not be
accepted acritically. Most likely
the expert would mistrust the data analysis, or the data themselves.
But she would perhaps also analyze critically her
prior beliefs in order to understand on what they were really grounded
and how solid they were.
As a matter of fact, scientists are ready to
modify their opinion, but with some care,
and, as the famous motto says,
“extraordinary claims require extraordinary evidence”.
Since scientific priors are usually strongly based
on previous experimental information, the problem
of `logically merging' a prior preference summarized
by
and a new experimental
results preferring `by itself' (that is when the result
is dominated by the `likelihood'
- see Sec.
),
summarized as
(or
, depending on
)
is similar to that of `combining apparently incompatible results.'
Also in that case, nobody would acritically
accept the `weighted average'
of the two results which appear to be in mutual disagreement.
A so called `skeptical combination' should be preferred,
which would even yield a multi-modal distribution [13].
This means that in a case like those of Fig.
the expert could think that either
- she is right, with probability
,
and she would just stick to her prior
;
- she is wrong, with probability
,
and she would switch to the posterior
provided by the likelihood alone, let us indicate it with
.
Therefore the degrees of belief of
will be
described by
. As far as we understand
from our experience she would hardly believe
the result obtained, `technically', plugging
her prior in the formulae - and we keep repeating
once more
Laplace's dictum that
“probability is good sense reduced to a calculus”.
In order to make our point more clear, let us look into the details
of the situation depicted in Fig.
with the help of Fig.
,
in which
Figure:
Closer look at the effect of the prior
Beta
shown in Fig.
.
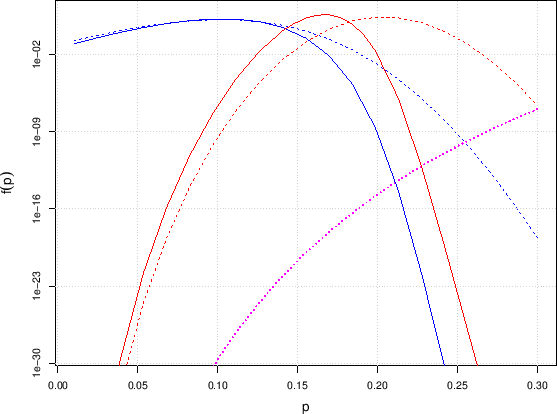 |
is reported in log scale, and the abscissa limited to the
region of interest. The blue curves, which are dominant below
, represent the posteriors obtained by a flat prior
(solid for
and
;
dashed for
and
).
Then, the dotted magenta curve is the tail at small
of the
prior
Beta
, which prefers values of
around
.
Then the red curves (solid and dashed as previously)
show the posterior distributions obtained by this new prior.
The shift of both distributions towards the right side
is caused by the dramatic reshaping due to
prior in the region between
and
in which
Beta
varies by
about 25 orders of magnitudes (!).
The question is then that no expert, who believes a priori that
should be most likely in the region between
0.5 and 0.7 (and almost certainly not below 0.40-0.45),
can have a defensible, rational belief that values
of
around 0.3 are
times more probable
than values around 0.1. More likely, once she has to give
up her prior, she would consider small values of
equally likely. For this reason - let us put in this way
what we have said just above - she will be in the situation
either to completely mistrust the new outcome, thus keeping her
prior, or the other way around.
The take-away message is therefore just
the (trivial) reminder
that mathematical models are in most practical cases
just dictated
by practical convenience and should not been taken literally in their
extreme consequences, as Gauss promptly commented on
the “defect” of his
error function immediately after he had derived it [9].
Therefore our addendum
to Laplace's dictum reminded above is don't get fooled by math.