For completeness, let us also try to get the closed expressions
of
and
, although only under the assumption
of a flat prior of
. In this case this choice is forced
from the fact that
cannot be expressed in term of a conjugate prior
which would then simplify the calculations. For the general case, in fact,
we have to change methods, moving to Markov Chain
Monte Carlo (MCMC), as done e.g.
in Ref. [1] and as it will be sketched in the next section.
In order to get the pdf of
, we need to restart from
the unnormalized joint distribution (
),
proceeding then like in Eq. (
),
but this time integrating over
and
and absorbing the constant priors in the proportionality factor:
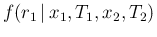 |
 |
d d |
(108) |
|
 |
d d |
(109) |
|
 |
d d |
(110) |
|
 |
d |
(111) |
|
 |
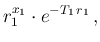 |
(112) |
thus reobtaining, besides normalization,
Eq. (
).
Similarly, we have
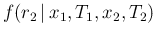 |
 |
d d |
(113) |
|
 |
d d |
(114) |
|
 |
d |
(115) |
|
 |
d |
(116) |
|
 |
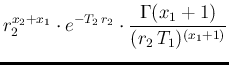 |
(117) |
|
 |
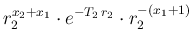 |
(118) |
|
 |
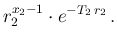 |
(119) |
We see that, differently from
,
the power of
is, instead of
,
,
that is we get an effect similar to that found for the
distribution of
. As a consequence, expected values
and standard deviation of
are
and
, respectively.