Probabilistic Inference and Forecasting in the Sciences
lectures to PhD students in Physics (40o Ciclo)
(Giulio D'Agostini and
Andrea Messina)
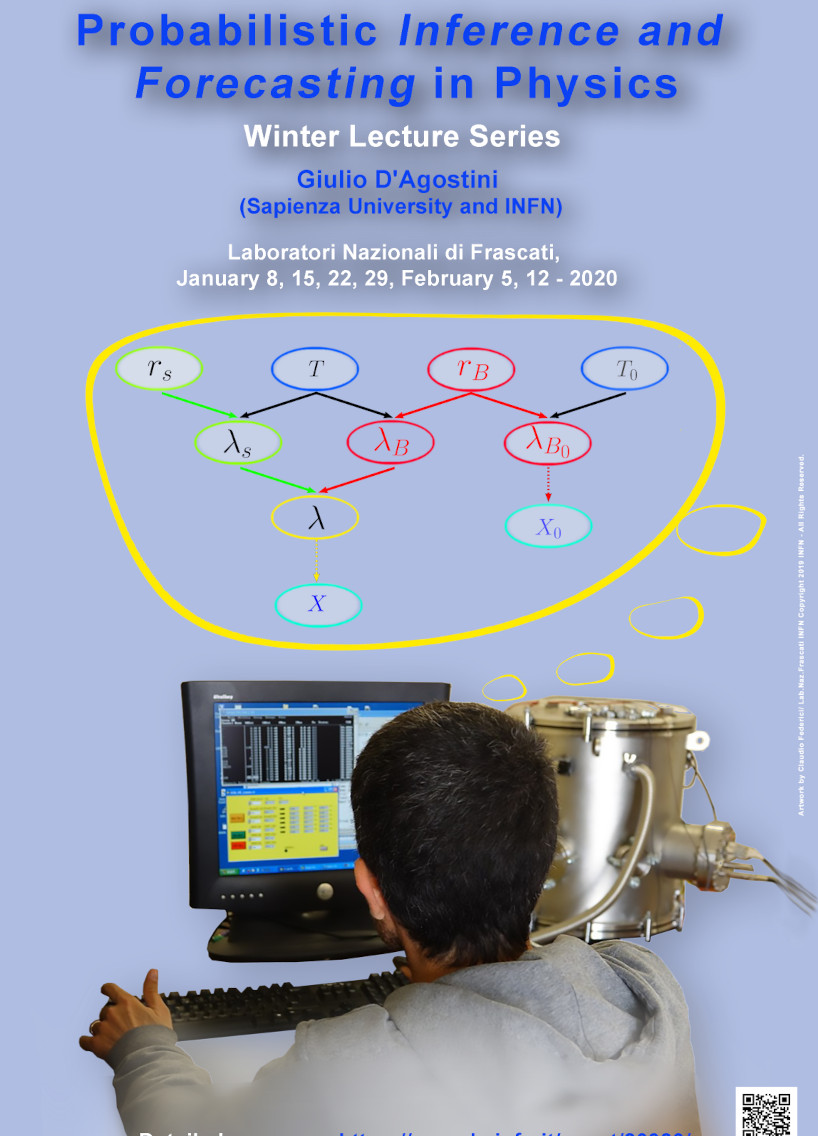
[Poster for lectures at LNF]
The course will be of about 40 hours (6 credits),
starting on Monday 13 January
[Il corso può essere frequentato, oltre che dai dottorandi,
anche da cultori della materia (da studenti della magistrale
a post-doc).
→ Gli interessati sono pregati di contattare i docenti.]
Contents
Time table
- Lecture 1 (13 January)
- Introduction to the course and entry test
in particular (although at qualitative level)
- models, model parameters ('μ', 'λ', 'p', etc.)
and empirically observed quantities ('x');
- measurement as a probabilistic inferential problem:
- ranking in probability the possible numerical values of the model parameters.
- Probabilistic inference and probabilistic forecasting
- A paradigm shift in data analysis from the old `formulae oriente approach'.
- Discussion (mainly qualitative) on some of the problems: nr. 1, 7 and 15.
References, links, etc.
- Lecture 2 (17 January)
-
- Continuing with the entry test:
→ discussion (mainly qualitative) on some of the problems: nr. 3, and 4.
- What we di when we do measurements
- from 'observed' values on instruments
... to the values of physical quantities;
- measurement models.
- Sources of uncertainty
- ISO/GUM dictionary,
— in particular, error ≠ uncertainty (!!)
— some details on source nr. 5, with some 'related' topics
of hystoric interest
— (see links and references below
— Physics facts and ideas are often interconnected!)
- Type A and
Type B uncertainties
- 'Usual' (old style) handling of uncertanties.
- A simple case (related to nr. 3 of the entry test):
inferring a physical quantity
associated with the parameter μ of a Gaussian
error distribution,
having observed a large sample sample
and assuming that
- μ does not change during the measurements;
- there are not 'systematic errors' (the only source
of uncertanty is nr. 10 of the GUM list).
References, links, etc.
- Lecture 3 (20 January)
-
- Probabilistic statements of the numerical value physical quantities
vs confidence intervals
and coverage
(somehow qualitative, as in invitation to think about and, as far
the 'frequentistic' terms are concerned,
to look in the preferred
books and lecture notes).
- Causes → Effects, and back.
- Simple example with two Causes and two effects (AIDS test).
- P(A|B) vs P(B|A).
- 'Prosecutor fallacy' (aka base rate fallacy,
base rate neglect and base rate bias).
- What is 'statistics'? [→"Lies , dammn lies and statistics"]:
descriptive statistics, probability theory, inference.
- "Lies, damned lies and... Physics":
claimes of discoveries based on 'p-values' ('n-σ')
References, links, etc.
- GdA, About the proof of the so called exact classical confidence intervals. Where is the trick?,
arXiv:physics/0605140 [physics.data-an]
- GdA, Probably a discovery: Bad mathematics means
rough scientific communication,
arXiv:1112.3620 [physics.data-an]
Please read, for the moment, only pp 1-9 and pp 20-21.
- An `important' case
(1997, appearing in GdA curriculum as antipublication)
- Other old (2000,2001) claim of discoveries based on 'sigmas',
one of which even concerned the Higgs particle (old style slides):
(Much time after the 1984 de Rujula's
Cemetery of Physics,
and many more claims have followed...)
- Lecture 4 (22 January)
-
- Probability of hytheses vs 'classical' hypothesis tests
- More on χ2: see last slide of previous lecture (updated)
- Mechanism behind the 'classiical' hypothesis tests:
- from falsificationism to p-values
... and misundestandings(!!)
- Doing logical mistakes and crimes with p-values:
- Examples of p-values based on χ2
References, links, etc.
- GdA, Bayesian reasoning in high energy physics,
Principles and applications.
Yellow Report CERN 99-03
For the moment:
- Chapter 1;
- Sections 2.1-2.6;
- Sections 3.3-3.5 (we shall come back to the 'details').
- GdA, "From Observations to Hypotheses: Probabilistic Reasoning Versus Falsificationism
and its Statistical Variations",
arXiv:physics/0412148 [physics.data-an],
only pp. 1-9, for the moment.
- GdA, Scoperte annunciate a colpi di 'sigma'
- "p-hacking,
or cheating on a p-value" (R-bloggers)
(much more on the web searching for
p-hacking
or data dredging))
- "If you torture the data long enough,
it will confess to anything" → immagini
- GdA, Probably a discovery: Bad mathematics means
rough scientific communication,
→ see related links here
and here
- R.L Wasserstein and N.A. Lazar,
"The ASA Statement on p-Values: Context, Process, and Purpose",
The American
Statistician, 70(2), 129–133
- M. Baker, "Statisticians issue warning over misuse of P values",
Nature, 531 (2016) 151.
- wiki/Misuse_of_p-values
(The link given in the slides does not longer exist, but the first items are
about the same)
- Significant, by xkcd
- Is Most Published Research Wrong?,
YouTube
by Veritasium (very well done!),
based on
- J. Bohannon, I Fooled Millions Into Thinking Chocolate Helps Weight Loss.
Here's How,
GIZMODO 27 May 2015
Some related material:
- For (just) an example, from Medical Science, on results reported
quoting p-values:(*)
- An `important' case
(1997, appearing in GdA curriculum as antipublication)
- Other old (2000,2001) claim of discoveries based on 'sigmas',
one of which even concerned the Higgs particle (old style slides):
(Much time ofter 1984 de Rujula's Cemetery of Physics,
and many more claims have followed...)
- Lecture 5 (24 January)
-
Per gli argomenti vedi slides
↠ Problemi
References, links, etc.
- Lecture 6 (27 January)
-
Per gli argomenti vedi slides
↠ Problemi
References, links, etc.
- GdA, The Gauss' Bayes Factor,
arXiv:2003.10878 [math.HO]
(See also here
for historical related issues, including
The Fermi's Bayes Theorem)
- GdA, Probability, Propensity and Probability of Propensities (and of Probabilities),
arXiv:1612.05292 [math.HO]
(more on the subject here and
here.)
- GdA, N. Cifani and A. Gilardi, Talking about Probability, Inference and Decisions. Part 1: The Witches of Bayes,
arXiv:1802.10432 [math.HO]
- Laplace A
Philosophical Essay on Probabilities
(prima traduzione italiana)
- Hugin:
Hugin Lite (free → download)
- Tutorials.
- Examples provided by the company:
Samples
- Ready-to-use models based on the six-boxes toy experiment:
- Try to edit the models
(within HUGIN), changing the probability
tables, adding nodes, etc..
- Try to write from scratch the (minimalist) model to solve
the AIDS problem, using the number suggested in the slides
for easy comparison.
just two nodes
- Infected, with two possible states,
Yes and No;
- Analysis result, with two possible states,
Positive/ and Negative.
- Modify the previous model, using equiprobable
priors for Infected/Non-Infected:
- compare the result with the those obtained
with (roughly) realistic priors;
- compare the result with the wrong one suggested
in the first lecture.
- Think then to the possible practical utility of
using equiprobable priors.
- Netica: a valid alternative to Hugin,
thanks also to the many available
whose interest goes beyond the specific package.
- S. Cenatiempo, GdA e A. Vannelli,
Reti Bayesiane: da modelli
di conoscenza a strumenti inferenziali e decisionali
- Lecture 7 (27 January)
-
Per gli argomenti vedi slides
↠ Problemi
References, links, etc.
- Lecture 8 (31 January)
-
Per gli argomenti vedi slides
↠ Problemi
References, links, etc.
- Lecture 9 (3 February)
-
Per gli argomenti vedi slides
↠ Problemi
References, links, etc.
- Dispense "Probabilità e incertezze di misura"
- GdA, Bertrand 'paradox' reloaded,
https://arxiv.org/abs/1504.01361
→ besides the 'paradox', the paper
contains details on exact tranformations of variables.
- GdA, Asymmetric Uncertainties: Sources, Treatment and Potential Dangers,
https://arxiv.org/abs/physics/0403086
- PDF of sum of two ('iid') asymmetric triangular distributions
done analytically using Mathematica:
[But it can be done more easily by MC, also extending it to many
triangulars,
using rtriang()
included in the script triang.R
available in the R web page]
- Write (pseudo-)random number generators for
- f(x) ∝ x (0 ≤ X ≤ 1)
- f(t) ∝ e-t/τ (0 ≤ T < ∞)
- f(x) ∝ cos(x) (-π/2 ≤ X ≤ π/2)
- X ∼ B10,1/5 (binomial distribution with n=10 and p=1/5)
[Note: in the cases 1, 3 and 4 use both techniques we have seen (lecture 7, pp. 17-18);
in the case 2 we can only use the "F-1(yR)" technique]
- Lecture 10 (7 February)
-
Per gli argomenti vedi slides
↠ Problemi
References, links, etc.
- As far as a JAGS is concerned (for the moment dont worry about its use
as inferential tool, but simply try to installe it and to run the basic examples):
(Much more can be found on the web, including
PyJAGS
and JAGS.jl)
- JAGS 'improperly' used as simple random generator:
In alternative, the model file can be defined inside the R script:
— simple_simulations_1.R
Moreovere, here is how to extract the individual histories and to make
customized graphics:
simple_simulations_graphics.R
(run the script after the previous one, and customize it at wish).
[During the lecture we have also seen how to add variables x5 and x6,
related to x1 and x2]
Notes for R users:
- the following packages need to be installed: rjags and coda
- the function 'pausa()' used in simple_simulations_graphics.R is given by
pausa <- function() { cat ("\n >> press Enter to continue\n"); scan() }
- Script waiting_2_counts.R (p. 36 of the slides)
Notes:
- expected value and standard deviation are evaluated in the script by numeric integration
- ... but we know the exact expressions.
- The curves, as well as the expected value and standard deviation,
can be also obtained using the app Probability Distributions, in which (pay attention!)
- the generic x stands for our time t;
- the rate (our r) is indicated by β;
- the number of counts (our k → c)
is indicated by α.
Updated script with some improvements: waiting_2_counts_gamma.R
- Lecture 11 (10 February
-
Per gli argomenti vedi slides
↠ Problemi
References, links, etc.
- Lecture 12 (14 February)
-
Per gli argomenti vedi slides
↠ Problemi
References, links, etc.
- Problema aggiuntivo sull'uso di JAGS
- la variabile 'lambda' è descritta da una gaussiana
di media 10000 e sigma 100;
- la variabile 'n' è descritta da una poissoniana avente
tale lambda (incerta)
- la variabile 'x' è descritta da una binomiale avente
tale 'n' incerto e p=0.95 ('certo').
- Variante del problema:
- si immagini che anche 'p' sia incerto e che sia
descritto da una Beta
avente valore atteso 0.95
e deviazione standard 0.05
[In entrambi i casi, sulla traccia degli esempi visti precedentemente,
valutare mediante MC le distribuzioni di probabilità
delle variabili incerte
e i riassunti statistici di interesse.]
- Lecture 13 (17 February)
-
Per gli argomenti vedi slides
↠ Problemi
References, links, etc.
- Basic MC's proposed last lecture
with remarks on the use of the Beta prior
- Simple examples of JAGS(*) (via rjags) for inference/forecasting:
- Binomial
→ Try, e.g., to change n0 and x0, keeping x0/n0 constant.
- Poisson
- Gaussian
(*) For the moment take it as a kind of black box.
We shall see in the following lectures how it works.
And, 'obviously', there is also the equivalent of 'rjags' for Python:
PyJAGS
(it seems there also
'something' for Julia)
↠ Working Python and Julia
versions of the above examples are welcome!
- Lecture 14 (21 February)
-
Per gli argomenti vedi slides
↠ Problemi
References, links, etc.
- Inference and prediction from a Gaussian sample (using JAGS/rjags):
- inf_mu_sigma_pred.R
— Which kind of prior does "dgamma(1.0, 1.0E-6)"
approximately model
— Modify the prior of of
τ=1/σ2 (*)
- still making use of a Gamma pdf;
- a) assuming an initial value of σ ≈ 10 ± 10 (mean and standard deviation);
- b) assuming an initial value of σ ≈ 1 ± 1 (mean and standard deviation).
[(*) For a better comparison of the results
it is recommended to split code in the
several scripts:
- one to simulate the data
- others to analyze the same data using
different models/priors. ]
[A proposito dei diversi significati della parola italiana statistica,
vedi qui]
- More on confidence intervals
- A proposito della previsione di una nuova media aritmetica
(vedi p. 7 slides di oggi),
ecco come la cosa veniva raccontata
in un libro(**)
dal simpatico sottotitolo.
[(**) questi sono solo esempi di quanto girava e gira ancora...]
- More on priors:
- For an introduction to MCMC, Metropolis and Gibbs sampler:
C. Andrieu et al., An introduction to MCMC for Machine Learning,
Machine Learning, 50 (2003) 5-43,
https://doi.org/10.1023/A:1020281327116
(local copy)
- The examples of children and adults playing throwing stones are from
Statistical
Mechanics: Algorithms and Computations by
Werner Krauth,(***)
with the relevant pages readable in the Amazon preview
- backup screenshots:
1,
2,
3,
4,
5,
6,
7,
8,
9
[(***)Online course on the subject, with Krauth
as main instructor, available
on Coursera]
- Inferring μ and σ from a sample:
Lecture notes Probabilità e incertezze di misura,
Parte 4, Sec. 11.6
- R scripts concerning other MC issues, expecially MCMC:
-
- Lecture 15 (24 February)
-
Per gli argomenti vedi slides
↠ Problemi
References, links, etc.
- GdA, Bayesian inference in processing experimental data:
principles and basic applications,
Sec. 9 (+ 1-5, as a summary)
(Preprint: arXiv:physics/0304102)
- Concerning the BUGS project, to which JAGS is somehow related
- R scripts (some using JAGS)
- Suggested variation of the script left as exercise:
- Extend the model in order to include
also the inference
of λ from which n derives
[Note: since n depends on the uncertain node λ,
the lower limit provided by ' I(nmin,)' is not needed
and not accepted by JAGS!]
- BAT
- Lecture 16 (28 February)
-
Per gli argomenti vedi slides
↠ Problemi
References, links, etc.
- GdA, CERN Yellow Report 99-03
(local copy of Part 2);
- Sec. 5.6.1-5.6.4
- Sec. 6.3.1-6.3.4
norm_sist_z.R
- Concerning model comparison and Ockham's razor
- D.J.C. MacKay, Chapter 28 of
Information Theory, Inference, and Learning Algorithms
(great book,
freely available)
- I. Murray and Z. Ghahramani,
A note
on the evidence and Bayesian Occam’s razor
- C.E. Rasmussen and Z. Ghahramani,
Occam’s Razor
- P. Astone, GdA and S. D'Antonio,
Bayesian model comparison applied to the Explorer-Nautilus 2001 coincidence data,
Class. Quant. Grav. 20 (2003) 769,
arXiv:gr-qc/0304096
- Introducing fits (just parametric inference)
GdA, Fits, and especially linear fits, with errors on both axes,
extra variance of the data points and other complications,
arXiv:physics/0511182 [physics.data-an],
Sec. 1-3
- GdA, Ratio of counts vs ratio of rates in Poisson processes,
arXiv:2012.04455 [stat.ME]
(only general ideas — details out of program)
- Lecture 17 (5 March)
-
Per gli argomenti vedi slides
↠ Problemi
References, links, etc.
- GdA, Checking individuals and sampling populations with imperfect tests,
arXiv:2009.04843
- GdA, What is the probability that a vaccinated person is shielded from Covid-19?,
arXiv:2102.11022
- GdA, Ratio of counts vs ratio of rates in Poisson processes,
arXiv:2012.04455
- GdA, CERN Yellow Report 99-03
- GdA, Fits, and especially linear fits ...,
arXiv:physics/0511182 ,
Secs. 1-2.
- GdA, Fit, in Italian,
(pdf version: Capitolo 12)
- GdA, Learning ... by playing with multivariate normal distributions
arXiv:1504.02065,
Sec. 10
- R scripts:
Suggested work:
- modify the script in order to:
- evaluate and plot also μy(xf)
-
modify further the script in order to:
- consider two extrapolations, one at xf1=30
and the other at xf2=32:
→ make the two plots, evaluate expected values and variances;
→ draw the scatter plot and evaluate the correlation
coefficient of yf(xf2) vs yf(xf1).
- Lecture 18 (19 March)
-
Per gli argomenti vedi slides
↠ Problemi
References, links, etc.
- Lecture 19 (2 April)
-
Per gli argomenti vedi slides
References, links, etc.
- V. Blobel, Least square methods
(local copy)
- GdA, On the use of the covariance matrix to fit correlated data,
https://inspirehep.net/literature/361137
- GdA, Confidence limits: what is the problem? Is there the solution?.
See also
- GdA and G. Degrassi, Constraints on the Higgs Boson Mass
from
Direct Searches and Precision Measurements,
arXiv:hep-ph/9902226;
- P. Astone and GdA, Inferring the intensity of Poisson
processes at limit of the detector sensitivity
(with a case study on gravitational wave burst search)
arXiv:hep-ex/9909047;
- Chapter 13 of Bayesian Reasoning in Data Analysis
— A Critical Introduction.
- GdA, A Multidimensional unfolding method based
on Bayes' theorem,
Nucl.Instrum.Meth.A 362 (1995) 487-498
(iNSPIRE HEP entry)
→ related material
- GdA, Improved iterative Bayesian unfolding,
arXiv:1010.0632
→ related material
- R/JAGS scripts:
Back to G.D'Agostini - Teaching
Back to G.D'Agostini Home Page